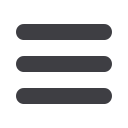
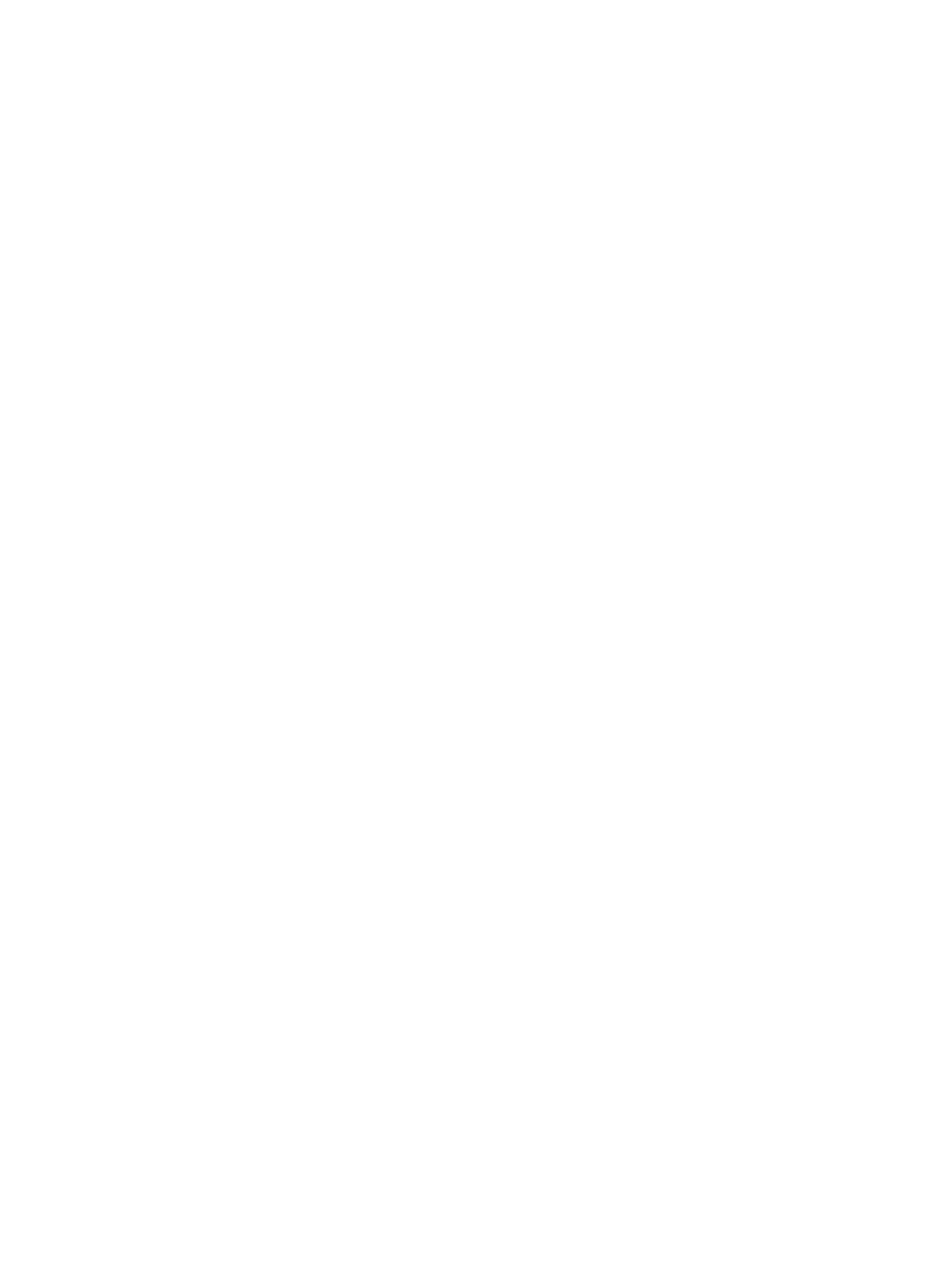
detected by all media. A total of 40, 48, 47 and 43 CPE were detected
on MacI, MacD, MacC ESBL and TSB broth respectively. Sensitivity
was highest for MacD (96%) (94%). Specificity was maximum for
MacI (97%).
The performance of media varied with carbapenem MIC and
carbapenemase gene associated with CPE isolate.
Discussion and/or Conclusion(s):
No single method is 100% sensitive
and specific for CPE detection. The choice depends on type of carba-
penemase gene prevalent locally. Combination of media increases the
sensitivity and specificity.
ID: 5033
Spread of hospital-acquired infections: a comparison of patient
transfer networks
Narimane Nekkab
1
, Pascal Astagneau
2
, Pascal Crépey
3
.
1
Conservatoire
National des Arts et Metiers, Ecole des Hautes Etudes en Sante Publique,
2
Centre de Coordination pour la Lutte Contre les Infections Associées aux
Soins,
3
Ecole des Hautes Etudes en Santé Publique
Background:
Hospital-acquired infections (HAIs), including emerging
multi-drug resistance organisms, continue to threaten all healthcare
systems.
Aim(s)/Objective(s):
To better understand how to reduce the scale
of HAI epidemic spread we compared and explored the characteristics
of patient transfer patterns in the French healthcare network to
identify their potential role in epidemic spread of HAIs within the
network.
Method(s):
Three patient transfer networks were compared: twowith
only HAI related patients (a specific and a more sensitive definition),
and all transferred patients. We assessed network characteristics,
computed centrality, community measures, and compared these
networks to Erdos-Renyi random models and to networks with
randomly selected patients.
Results:
More than 10 million patient transfers were recorded in
France in 2014 for 2.3 million patients, building a network of 2063
hospitals and 50026 connections. The network of specific-HAI patients
was composed of 1266 hospitals and 3722 connections for 13627
patient transfers, while the suspected-HAI network was composed of
1975 hospitals and 18812 connections for 128681 patient transfers.
These networks displayed a scale-free structure for hospital
’
s number
of connections (in and out), and transfers. They also feature a small-
world characteristic. Communities within the networks had small
average inner distances between 22km to 88km, depending on the
modularity of the community detection algorithms.
Discussion and/or Conclusion(s):
These characteristics may have a
tremendous effect on the diffusion of nosocomial pathogens. In
addition, different patient inclusion criteria could impact network
characteristics and the identification of key hospital centres, patient
flow trajectories, and regional clustering that may serve as a basis for
novel wide-scale HAI control strategies.
ID: 5035
Use of ICD-10 coding in the hospital setting for infectious disease
and antimicrobial resistance surveillance
Meghan Perry
1
, Catriona Parker
2
, Oliver Koch
2
, Mark Woolhouse
3
.
1
NHS Lothian and University of Edinburgh,
2
NHS Lothian,
3
University of
Edinburgh
Background:
Databases of hospital admissions using the International
Classification of Disease codes could be a valuable source of infectious
diseases surveillance.
Aim(s)/Objective(s):
This study
’
s aim was to assess the accuracy of
ICD-10 codes for infectious diseases diagnoses and recording of
antimicrobial resistance.
Method(s):
The ICD-10 codes from 100 discharges in the Regional
Infectious Diseases Unit, Western General Hospital, Edinburgh starting
on June 1
st
2015 were recorded. Corresponding discharge letters were
reviewed to verify the accuracy of the codes. Microbiological records of
the admission were checked to confirm that all relevant information
had been recorded on the discharge letter.
Results:
Sixty-eight discharges had appropriate ICD-10 codes relating
to the discharge letter. Of the 32 inappropriate codes 21 were incom-
plete, 8 were inaccurate, 2 were unconfirmed diagnosis and one
diagnosis had not been coded. On reviewing the microbiology 16
results were unrecorded in discharge letters that would impacted the
ICD-10 codes. Sixteen of the pathogens identified were resistant to one
or more antibiotics. However, only 2 of the discharge diagnoses
mentioned antimicrobial resistance and no ICD-10 resistance codes
were used.
Discussion and/or Conclusion(s):
Although a small sample size, this
audit highlights that the use of hospital ICD-10 databases for
epidemiological surveillance of infectious diseases diagnoses and
antimicrobial resistance would not currently give an accurate picture.
There are areas where the ICD code format could be improved to allow
for adequate representations of infectious diseases diagnoses includ-
ing recording of the clinical impact of antimicrobial resistance.
Increased awareness in doctors of the coding process and education
of the coders is recommended.
ID: 5036
Computer-assisted risk assessment of hospital infections: a
preliminary implementation in Polish hospitals
Andrzej Jarynowski
1
, Damian Marchewka
2
, Andrzej Grabowski
3
.
1
Jagiellonian University,
2
Interdisciplinary Research Institute in Wroclaw,
3
CIOP
–
National Research Institute in Warsaw
Background:
We create an intuitive and functional desktop
application (free of charge, open licensed) to support the work of
the hospital epidemiologists in preventing and containing hospital
infections.
Aim(s)/Objective(s):
The goal of the application is reconstructing the
most likely paths of infection and classifying places and individuals
into different risk groups. We study the time-varying structure of
contacts reconstructed from the data sets collected in Polish and
Swedish hospitals. We will apply theory of temporal networks to
improve computer-assisted hospital infection analyses.
Method(s):
The algorithm processes data from the register of patient
admissions and discharges from each hospital unit (wards, clinics,
etc.), microbiological laboratory test results and medical staff
register. The patients
’
structure of contacts (persons who had
visited the same care facility) and functional paths are reconstructed.
Epidemiological models are implemented on a temporary network
of contacts (where each link can provide a path for the pathogen
transmission).
Results:
In a preliminary setup, we analysed only one alarm pathogen:
MRSA. With simulated infection paths, we were able to compute
network measures for patients. We obtained the risk of getting
infected, based on the patient
’
s incoming connections, and the risk of
spreading infections resulting from outgoing connections (both
analogous to Google algorithm).
Discussion and/or Conclusion(s):
We provide a costless preventive
computer-assisted tool against HAI. The biggest effort from hospital
administration is mapping hospital topology, entered manually by a
hospital employee. Later on, data is imported from hospital informa-
tion system. The results of the algorithms are presented
‘
real-time
’
to
an hospital epidemiologist for interpretation and applying preventive
control.
ID: 5058
Invasive pneumococcal disease in the North East of England: Rising
incidence in 2014/2015 and 2015/2016
Kate Houseman, Gareth J Hughes, Kaye Chapman, Russell Gorton,
Deb Wilson.
Public Health England
Background:
A substantial and sustained increased incidence of
invasive pneumococcal disease (IPD) has been observed across the
North East of England (NEE) fromApril 2014, reversing the decline that
Abstracts of FIS/HIS 2016
–
Poster Presentations / Journal of Hospital Infection 94S1 (2016) S24
–
S134
S128